A Guide to Data Auditing to Transform Your Business Data into Strategic Assets
Discover how systematic data auditing can reduce the annual cost of poor data quality, streamline pipeline creation, and turn your data into trusted insights that drive confident decisions and competitive advantage.
Companies are drowning in data, yet starving for insights. Without effective data auditing, raw numbers flood their systems while they struggle to extract any real value. This costs them dearly—poor data quality drains millions yearly from company bank accounts and leads to flawed decisions.
According to Gartner research, organizations believe poor data costs them an average of $15 million per year—a problem that effective data auditing can help mitigate. Beyond financial costs, bad data erodes trust in analytics and freezes decision-making when quick action matters most.
Data auditing bridges the gap between raw information and strategic assets. By regularly checking data quality, integrity, and usage, companies transform scattered data points into trustworthy insights that give them an edge.
In this article, we explore data auditing, its benefits, key components of an effective auditing framework, and practical steps to implement a comprehensive data audit in your organization.
What is data auditing?
Data auditing is a systematic process of evaluating the quality, reliability, accessibility, and governance of an organization's data assets. It goes beyond simple IT security checks, focusing on the strategic value and business utility of data. This comprehensive examination spans multiple dimensions, including completeness, accuracy, consistency, timeliness, and relevance.
When conducting a data audit, organizations scrutinize various elements, including data sources, transformation processes, access controls, and usage patterns, to see how data is being used across the organization.
Benefits of data auditing
According to our survey, 45% of organizations report that ensuring data quality and accuracy is their top data processing challenge.
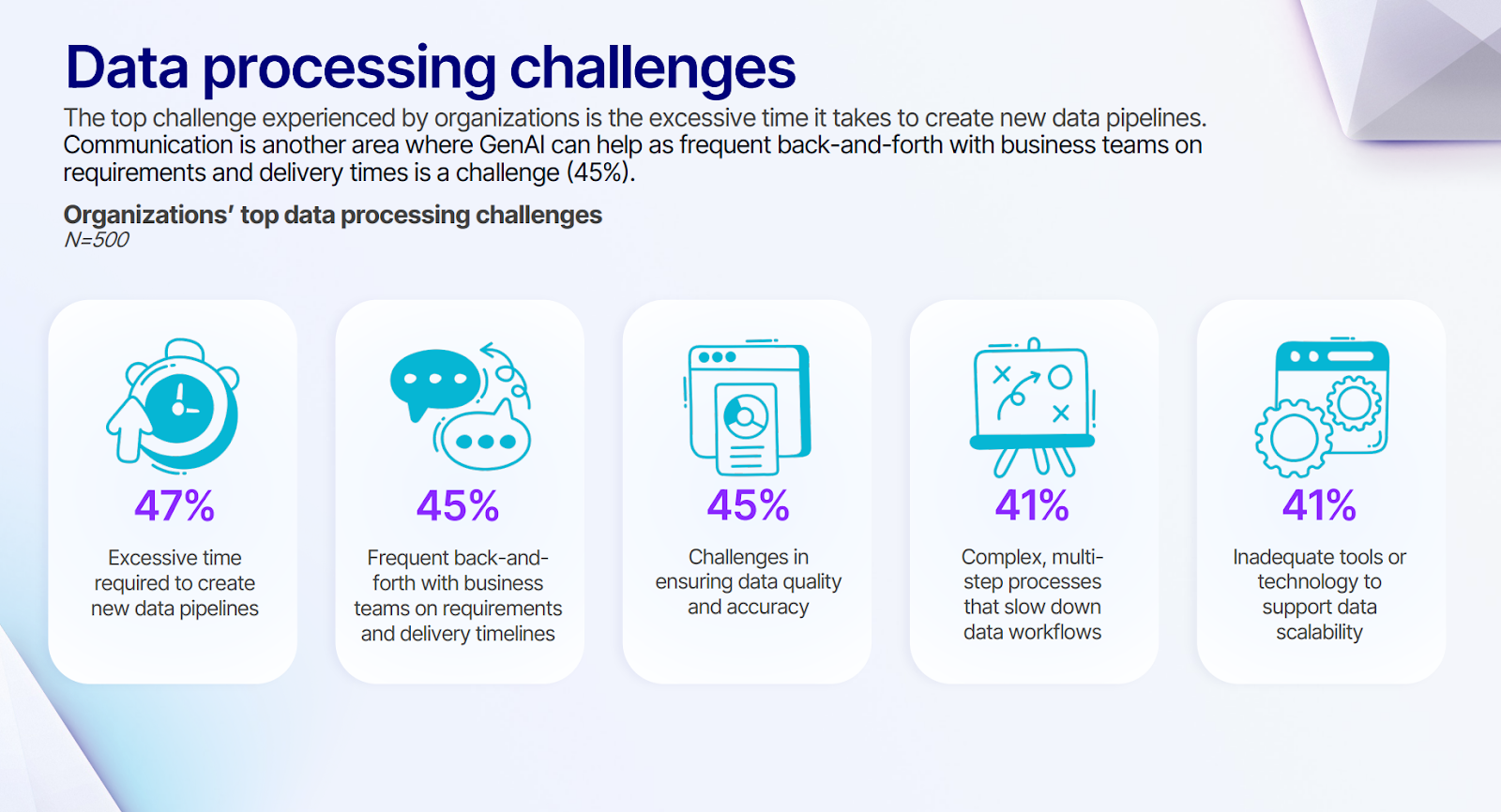
These findings underscore the critical need for systematic data auditing processes that can address quality issues upstream while streamlining pipeline development. As data grows more complex and businesses demand faster insights, robust data auditing becomes even more critical. Regular data auditing delivers real benefits:
- Better decisions: Clean, accurate data leads to reliable insights and confident decision-making at all levels.
- Lower costs: Finding and fixing data redundancies and errors streamlines processes and reduces data management expenses.
- Regulatory compliance: Regular audits help you stay ahead of regulations, reducing penalty risks and protecting your reputation.
- Happier customers: Accurate customer data enables more personalized interactions, boosting satisfaction and loyalty.
- Trust in analytics: When people trust the data, they actually use and act on insights.
- Risk reduction: Audits uncover potential weaknesses in data handling, allowing proactive management.
- Streamlined operations: Standardized data across systems reduces errors and speeds up processes that use multiple data sources.
- Strong governance: Audits provide the foundation for developing and refining data policies and practices.
By addressing these areas, data auditing creates a solid foundation for data-driven decisions, operational excellence, and competitive advantage.
The relationship between data auditing and data governance
Data auditing and data governance work hand-in-hand. Auditing provides evidence that drives effective governance, while governance creates the framework, making auditing meaningful and actionable.
Furthermore, modern data governance sets rules, policies, and standards for handling data, defining roles, and management processes. Data auditing verifies if these measures are being followed and how well they work.
Regular audits reveal governance gaps and identify new risks requiring updated policies. This feedback loop helps organizations continuously refine their governance frameworks to stay relevant.
A major challenge companies face is the "which report is correct?" problem, where departments present conflicting numbers from inconsistent data sources. This issue often stems from data silos within the organization. Overcoming data silos is crucial to ensuring consistency and accuracy across the organization, thereby strengthening trust in business intelligence.
Systematic data auditing enables the creation of a "single source of truth" that builds organization-wide confidence. By verifying data quality and consistency, audits ensure all departments work with reliable information and can agree on standards, like definitions of metrics, speeding up decision cycles and allowing teams to focus on strategic actions rather than questioning numbers.
When stakeholders trust data, they invest in data-driven initiatives, creating a virtuous cycle where better data quality leads to better decisions. Similarly, data auditing provides the foundation for safe data democratization, creating guardrails that allow business users to access data independently without creating quality or compliance risks.
Key components of an effective data auditing framework
A robust data auditing framework helps organizations ensure data quality, compliance, and strategic value. By focusing on critical components, businesses can create a comprehensive approach balancing technical requirements with organizational processes.
Data quality assessment
Data quality assessment forms the core of any effective auditing framework, evaluating crucial dimensions including accuracy, completeness, consistency, timeliness, validity, and uniqueness. This assessment applies to both structured and unstructured data.
An effective assessment ensures data correctly represents real-world values, contains all required information, maintains uniformity across systems, remains current and relevant, follows defined formats and business rules, and contains no duplicate records.
Organizations typically employ a combination of automated profiling tools to identify anomalies and patterns, statistical sampling methods to efficiently assess large volumes of data, and business rule validation to ensure alignment with operational requirements. By connecting quality metrics directly to business impact, organizations transform technical exercises into strategic drivers.
For example, incomplete customer information directly impacts marketing campaign effectiveness, while inaccurate financial data leads to flawed forecasting and resource allocation. Context-specific quality definitions are essential, ensuring standards align with business needs across various departments and use cases.
When implemented systematically, quality assessments build the foundation for trustworthy analytics and confident decision-making throughout the organization.
Data pipeline integrity
Data pipeline integrity focuses on maintaining trust in the processes that transform raw data into actionable insights. Shehzad Nabi, Chief Technology Officer at Waterfall Asset Management, notes how the integrity of data pipelines represents the hidden infrastructure of modern analytics. Without rigorous validation at each transformation stage, even the most sophisticated analysis will produce misleading results.
Comprehensive pipeline audits, supported by effective monitoring features in ETL tools, examine hidden transformations that might alter data without clear documentation, gaps in error handling that could cause information loss or corruption, and complex dependencies between interconnected data flows and systems.
Beyond technical elements, effective integrity assessments evaluate code quality to ensure efficient and well-documented transformations, performance metrics to identify processing bottlenecks, testing practices to validate robust validation processes, and deployment controls to prevent unauthorized changes that might compromise data quality. Adopting a modern data pipeline architecture can further enhance data quality and integrity.
By maintaining pipeline integrity through systematic auditing, organizations establish clear data lineage, creating transparency from source systems through to analytics platforms. This visibility enables better troubleshooting when issues arise, supports compliance requirements for data provenance, and builds stakeholder confidence in downstream analytics.
Data usage and value analysis
Data usage and value analysis transcends traditional quality checks to assess how effectively data assets create tangible business value across the organization. This component examines utilization patterns to identify datasets critical for decision-making, uncovers underutilized information assets with untapped potential, and evaluates the return on investment for various data resources to guide strategic investments.
By quantifying data asset value, organizations can focus governance efforts where they matter most, justify infrastructure investments based on measurable outcomes, and align data strategy directly with business objectives. Leveraging data integration insights ensures that data assets are effectively utilized.
The insights gained from usage analysis often reveal surprising patterns – for instance, a retail payment network might discover that combining point-of-sale data with social media sentiment provides significantly more accurate sales forecasts than either dataset alone, driving targeted investments in integration capabilities.
Data-driven companies consistently outperform peers in customer acquisition and retention by systematically analyzing which data delivers the greatest competitive advantage.
This perspective transforms data from a technical concern into a strategic asset, enabling organizations to prioritize initiatives that maximize business impact while eliminating investments in data that doesn't contribute meaningfully to organizational goals.
Tools for modern data auditing
With increasing data volumes and complexity, using the right tools for data auditing has become crucial. Modern solutions offer automation, analytics, and integration capabilities that streamline audits, improve accuracy, and ensure compliance.
Specialized audit solutions
Some organizations choose to onboard a dedicated audit solution. Platforms leading the field include:
- ACL Robotics: Known for robust automation of data auditing and risk assessment. Particularly effective for large-scale analysis and repetitive audit tasks.
- TeamMate+ Audit: Offers a comprehensive platform for audit management, from planning to reporting. Its centralized dashboard and customizable workflows help teams standardize processes.
- SAP Audit Management: Tailored for large, regulated organizations, providing integrated risk assessment and audit lifecycle management.
- DataSnipper: Focuses on intelligent automation to maximize audit efficiency. Users experience efficiency gains during audit periods through automated data matching and validation.
Gartner's review of audit management solutions highlights additional options like Onspring, offering no-code process automation for connecting data, teams, and processes.
Audit capabilities in modern data platforms
Your organization does not necessarily need a dedicated solution for data auditing. Modern cloud data platforms like Databricks are increasingly embedding audit functionality directly into their tools. This approach offers seamless integration with existing data operations, providing comprehensive monitoring and governance capabilities.
Databricks provides robust auditing capabilities through its Unity Catalog and Delta Sharing features. This enables organizations to monitor data access, usage patterns, and data sharing activities, ensuring effective data governance and security.
Unity Catalog delivers detailed audit logs of user activities, including workspace access and account-level operations, all accessible through system tables with sample queries provided for analysis.
Delta Sharing extends these capabilities to external data sharing, logging who accesses which tables and what queries they run. This level of detail helps organizations track data lineage and maintain accountability across their data ecosystem.
With built-in quality controls, testing features, and data discovery tools, Databricks creates a comprehensive audit framework that supports both internal and external data operations.
These integrated capabilities represent the future of data auditing, where audit controls are built directly into data pipelines rather than applied as separate processes.
Steps to conduct a comprehensive data audit
A comprehensive data audit requires a structured approach to ensure thorough evaluation and actionable insights. Follow these steps to assess your data landscape, identify issues, and implement improvements effectively.
Plan your data audit
Define clear objectives and scope for your data audit. Identify key stakeholders and build a cross-functional team with the necessary expertise. George Mathew, Managing Director at Insight Partners, emphasizes how data auditing isn't just an IT function, but a business imperative. Prioritize data assets based on business impact, risk level, and strategic value to focus efforts where they matter most.
Next, categorize your data into analytical, operational, and customer-facing segments to determine the appropriate audit focus for each. Establish baseline metrics to measure progress throughout the audit process, such as data quality scores, compliance rates, or efficiency indicators.
Secure executive sponsorship early. Leadership buy-in is crucial for resources and driving change based on audit findings. Communicate the audit's importance in terms of risk mitigation, cost savings, and improved decision-making.
Afterward, set realistic timelines and milestones for each audit phase. Consider your organization's size, data complexity, and available resources when planning. Be ready to adjust your approach as you uncover new information during the audit.
The data audit process
Start by establishing data quality metrics and standards tailored to your organization's needs. Key metrics typically include accuracy, completeness, consistency, timeliness, and validity. Automated profiling tools can quickly assess large datasets against these criteria.
Next, collect and analyze your data. Use a mix of automated tools and manual inspection to evaluate your data assets. Look for common issues like NULL values, schema inconsistencies, duplicate records, and data type mismatches.
Focus on data lineage and transformation processes. Understanding how data flows through your organization helps identify potential failure points or quality degradation. Adopting a data mesh architecture can facilitate better data management and auditing processes. Document your findings meticulously, including the location of issues within your data ecosystem.
Calculate operational metrics like the number of data quality incidents, time to detection, and time to resolution. These metrics provide insights into your current data management practices and highlight improvement areas.
Assess the business impact of data quality issues. Quantify the potential revenue loss from inaccurate customer data or the compliance risk from inconsistent regulatory reporting. This information helps prioritize remediation efforts.
Post-audit actions
Transforming audit findings into a strategic improvement plan represents the critical bridge between assessment and value creation. Begin by developing a comprehensive remediation roadmap that clearly identifies ownership, realistic timelines, and measurable success metrics for each identified issue.
Prioritization should balance business impact, resource requirements, and dependencies between systems. Your plan should specifically identify impacted data tables, affected systems, user groups, and departments that rely on this data, and the criticality of related data pipelines. Consider upcoming projects that depend on clean data when sequencing improvements to maximize downstream benefits.
Communication emerges as a crucial success factor in this phase – develop tailored messaging for different stakeholder groups, emphasizing technical details for implementation teams while focusing on risk mitigation and ROI when addressing executive leadership. For end-users, highlight how quality improvements will enhance their daily workflows and decision-making capabilities.
Establishing ongoing monitoring mechanisms proves essential for maintaining momentum, with many organizations implementing data observability tools that provide continuous insights into quality and usage patterns.
This proactive approach catches issues early, preventing business impact and reducing remediation costs. Use audit insights to refine your broader data governance strategy by updating policies, procedures, and training programs based on patterns identified.
This creates an iterative improvement cycle that gradually builds a quality-focused data culture throughout the organization, transforming data auditing from a periodic assessment into a continuous enhancement engine driving competitive advantage.
Enabling data-driven excellence with integrated data auditing approaches
Data auditing shouldn't be isolated, or a one-off exercise, but an integral part of how data moves through your organization. By embedding audit controls directly into data pipelines, you maintain business agility while ensuring governance and compliance.
Prophecy bridges the gap between governance and self-service, letting teams build audit controls directly into their data workflows. Here's what makes Prophecy ideal for data-driven excellence:
- Visual pipeline building: Create and modify complex data pipelines using an intuitive drag-and-drop interface, making it easy to incorporate audit checkpoints without deep technical expertise.
- Automated lineage tracking: Gain end-to-end visibility into how data flows through your organization, simplifying audit trails and impact analysis.
- Customizable governance rules: Define and enforce data quality, security, and compliance rules directly within pipelines, ensuring consistent application of audit controls.
- Collaborative workflows: Enable cross-functional teams to work together on data projects, fostering a culture of shared responsibility for data quality and compliance.
- Scalable cloud architecture: Leverage Databricks’ cloud-native technologies to handle growing data volumes and evolving audit requirements without compromising performance.
To overcome the growing backlog of data quality issues that prevent organizations from fully trusting their data, explore How to Assess and Improve Your Data Integration Maturity to empower both technical and business teams with self-service quality monitoring.
Ready to give Prophecy a try?
You can create a free account and get full access to all features for 21 days. No credit card needed. Want more of a guided experience? Request a demo and we’ll walk you through how Prophecy can empower your entire data team with low-code ETL today.
Ready to see Prophecy in action?
Request a demo and we’ll walk you through how Prophecy’s AI-powered visual data pipelines and high-quality open source code empowers everyone to speed data transformation
Get started with the Low-code Data Transformation Platform
Meet with us at Gartner Data & Analytics Summit in Orlando March 11-13th. Schedule a live 1:1 demo at booth #600 with our team of low-code experts. Request a demo here.