Use Spark interims to troubleshoot and polish low-code Spark pipelines: Part 2
In Part 1, we learned an easy way to troubleshoot a data pipeline using historical, read-only metadata. Now, I want to dig in and polish my individual spark data frames.
In Part 1 we learned an easy way to troubleshoot a data pipeline using historical read-only metadata. Now I want to dig in and polish my individual spark dataframes (or RDDs). Here I have disabled column pruning temporarily so we can sample the data output from each dataframe.
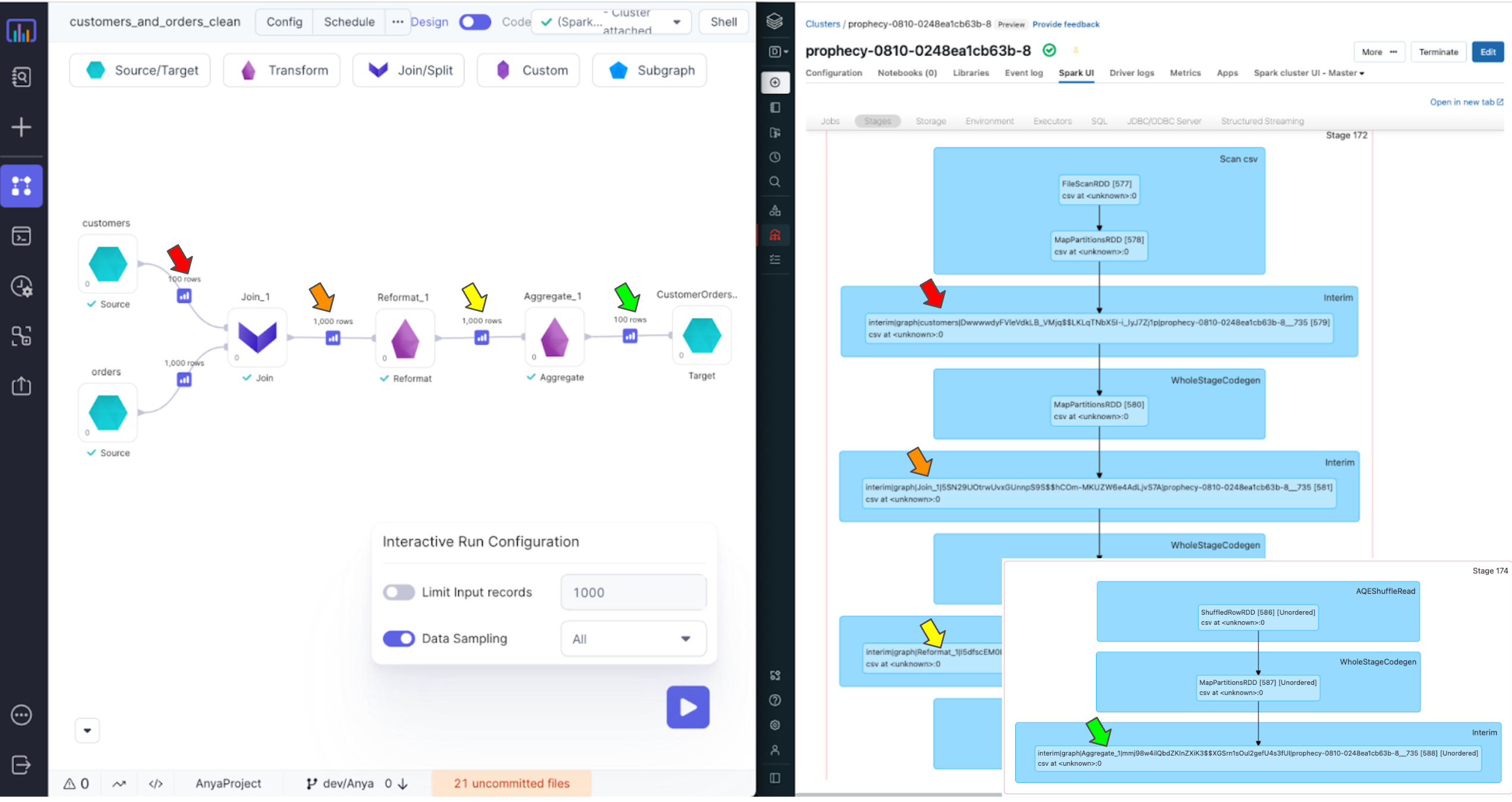
Let's see how the data pipeline could be improved. Interims show me some sample data for each step of my pipeline. Let's iterate
Now I understand how my individual dataframes behave and I’m happy with my pipeline. As usual, I can view my pySpark code changes and push them to my git repo.
Interim data sampling makes my troubleshooting easier - I can conceptualize the visual flow, compare historical runs (see Part 1 of this blog), and inspect individual dataframes ALL in a low-code interface for Spark. Finally, spark has a visual IDE!
How can I try Prophecy?
Prophecy is available as a SaaS product where you can add your Databricks credentials and start using it with Databricks. Or you can use an Enterprise Trial with Prophecy's Databricks account for a couple of weeks to kick the tires with examples. We also support installing Prophecy in your network (VPC or on-prem) on Kubernetes. Sign up for your 14 day free trial account here.
Ready to give Prophecy a try?
You can create a free account and get full access to all features for 21 days. No credit card needed. Want more of a guided experience? Request a demo and we’ll walk you through how Prophecy can empower your entire data team with low-code ETL today.
Ready to see Prophecy in action?
Request a demo and we’ll walk you through how Prophecy’s AI-powered visual data pipelines and high-quality open source code empowers everyone to speed data transformation
Get started with the Low-code Data Transformation Platform
Meet with us at Gartner Data & Analytics Summit in Orlando March 11-13th. Schedule a live 1:1 demo at booth #600 with our team of low-code experts. Request a demo here.