Data Cataloging: A Strategic Growth Enabler for Scaling Business
Discover how data catalogs evolve beyond simple organization to become strategic business drivers—providing advanced search, AI-powered insights, and seamless integration that turns data chaos into a competitive advantage.
Data teams spend countless hours hunting for assets rather than extracting insights, while business users wait weeks for answers to seemingly simple questions.
Modern data catalogs have emerged as powerful strategic assets that extend far beyond simple data organization. These modern data catalogs transform businesses by turning information into decision-making fuel. They help companies stand out in the market and run better operations by changing how they use information to create value.
In this article, we'll explore how data cataloging transforms from a basic inventory function into a strategic business enabler that drives growth, market differentiation, and operational excellence.
What is a data catalog?
A data catalog is a centralized, metadata-driven system that serves as a comprehensive inventory and navigational tool for an organization's data assets. It acts as a single source of truth, enabling you to discover, understand, and trust data across the enterprise.
Modern data catalogs go beyond simple listings, providing rich context, quality metrics, and usage information that make data meaningful and actionable for both technical and business users.
Data catalogs bridge the gap between having data and actually using it effectively. They help companies turn raw numbers into valuable insights, leading to faster decisions and better results. By showing what data exists, where it came from, and how it connects to other information, data catalogs dramatically cut down the time you need to find and use relevant data.
Features of a data catalog
Modern data catalogs incorporate a range of powerful features that enable efficient data discovery, understanding, and governance. Here are the capabilities that form the foundation of an effective data catalog system:
- Advanced search capabilities allow you to quickly find relevant data assets using keywords, tags, or metadata attributes.
- Automated collection and organization of technical, business, and operational metadata provide context and clarity for data assets.
- Visual representation of data flows and transformations helps you understand data origins and impacts, facilitating self-service data preparation.
- Features like commenting, rating, and sharing enable knowledge sharing and collective data curation across teams.
- Seamless connections with various data sources, analytics tools, and data governance systems create a unified data ecosystem.
- Intelligent analysis of data content and structure helps identify data quality issues and patterns.
- Granular permissions and policy enforcement ensure data is accessed and used appropriately.
- A centralized repository of business terms and definitions promotes consistent understanding across the organization.
At the heart of these capabilities lies the concept of metadata, which provides the contextual information needed to make data meaningful and useful across the organization. Understanding metadata is crucial to fully leveraging the power of a data catalog.
What is metadata?
Metadata is structured information that describes and makes it easier to find, use, or manage your data assets. Metadata is all about "data about data".
There are three main types of metadata, each serving different purposes:
- Technical metadata includes the nuts and bolts: schema definitions, data types, and formats—essentially the structural blueprint.
- Process metadata tracks the journey: where data came from, how it changed, and who used it.
- Business metadata is where human context lives: definitions, ownership, and why this data matters to the company. These three types work together to create a complete picture that helps people find, understand, and properly govern data across your organization.
Benefits of a data catalog
Here are the advantages of a data catalog:
- Users find relevant data quickly instead of wasting hours searching.
- Central management of data policies, access controls, and audit trails keeps you secure and compliant, facilitating modern data governance.
- Streamlined data processes reduce duplicate work and improve resource use.
- Knowledge sharing breaks down silos and helps everyone use data effectively, enhancing data literacy.
- Clear visibility into data origins and quality builds confidence in data-driven decisions.
- Data scientists and analysts get quick access to reliable, well-documented data.
- Less duplicate work and fewer IT requests for data access save money and time, reducing IT strain.
A good data catalog turns your complex data landscape into an organized, accessible resource that drives business value and gives you an edge over competitors.
Functions and use cases of data catalogs in data governance
Data catalogs bring data governance to life by providing visibility, control, and trust across your data landscape. They turn abstract governance policies into practical actions. Here's how data catalogs work in real governance situations.
Ensure data quality and consistency
Data catalogs shine a spotlight on quality issues across your organization. They display quality metrics, flag problems, and document validation rules so everyone sees the same quality picture.
Tools like data profiling, quality scoring, and issue tracking help organizations catch problems before they affect decisions. It's the difference between preventing errors and fixing them after they've caused damage.
Picture this: your data catalog flags inconsistent customer addresses across different systems, allowing your data team to standardize them before they cause problems. This ensures everyone downstream uses the same high-quality information.
By making quality visible, data catalogs help users make smart decisions about which data to trust. Analysts can quickly check if a dataset is reliable before building critical reports or models on it.
Support regulatory compliance initiatives
Compliance officers rely on data catalogs for ensuring compliance with regulations like GDPR, CCPA, HIPAA, and financial rules. They provide the documentation, lineage tracking, and usage logs that auditors demand.
Key compliance features include:
- Sensitive data identification
- Access logging
- Policy documentation
- Automated lineage tracking
These capabilities drastically cut compliance costs by automating documentation and evidence gathering. During an audit, your data catalog can instantly show where regulated data exists and how it's being used.
For example, a multinational company can use its data catalog during a GDPR audit to trace personal data across systems, show consent management, and provide a complete history of who accessed what data and when. This comprehensive view satisfied auditors and improved their overall governance.
Enable data stewardship and ownership
Data catalogs create clarity about who owns and is responsible for each data asset. This accountability improves quality and gives users a direct line to experts when they have questions.
Catalogs give stewards practical tools to manage their domains:
- Approval workflows
- Issue tracking
- Usage monitoring
These features help companies move from casual, ad hoc stewardship to structured programs that work across the entire organization, scaling data engineering in the process. For example, a large retailer can assign ownership of product data to the merchandising team and customer data to marketing through their data catalog.
This clear division of responsibility led to more accurate, timely updates of critical business information.
Facilitate data lineage and impact analysis
Data catalogs track information as it moves through systems, showing its origins, transformations, and uses in visual lineage graphs. This helps users understand where data came from, how it changed, and if it's right for their needs.
Impact analysis shows what downstream reports, dashboards, and applications would break if source data changed. This is crucial for making changes safely and maintaining reliable data processes.
For example, a financial institution can use lineage mapping in their data catalog to troubleshoot discrepancies in quarterly reports. By tracing the data back to its source, they found a calculation error in a middle system, fixed it, and corrected their financials.
Lineage also helps meet regulations requiring data traceability. Companies can prove the complete journey of sensitive information from collection to deletion, satisfying strict regulatory requirements.
These comprehensive governance capabilities help organizations build a culture where data is trusted and compliant. They turn governance from paper policies into practical, enforceable practices that make data more valuable and reliable.
Key components of effective data catalogs for business growth
Data catalogs have evolved beyond basic inventories into sophisticated knowledge platforms that capture both technical details and business context. They drive business growth by enabling faster insights, better collaboration, and smarter decision-making. Let's look at what makes data catalogs effective strategic tools.
Automated metadata collection and enrichment
Modern data catalogs use automation to discover and document data assets without manual effort. This automation is essential for accuracy at scale, especially when data changes quickly and manual approaches can't keep up.
Automated tools perform tasks like:
- Database schema scanning
- File system crawling
- API-based synchronization
- ML-based classification of data assets
These processes capture technical details and usage patterns automatically, while still needing human input for business context and quality assessments. An automated scanner might find a new database table and its structure, but you'll need a data steward to explain its business purpose and reliability.
Automation solves the biggest challenge: keeping data catalogs current. It ensures users always see up-to-date information about data assets. This timeliness builds trust in the catalog and supports data-driven decisions across your organization.
Enhancing business context through rich annotations
Good data catalogs go beyond technical details by capturing business context through business glossaries, tags, ratings, and user descriptions. This context turns technical metadata into information that helps users understand if data fits their needs, which is crucial for modern ETL processes.
Key features for adding business context include:
- Business glossaries defining common terms and metrics
- Tagging systems for categorizing data assets
- User ratings and reviews of datasets
- Collaborative annotation tools for subject matter experts
When experts share their knowledge about data definitions, quality, and appropriate uses, data catalogs become living repositories of organizational wisdom. This shared understanding improves decisions by helping users interpret data correctly and understand its limitations.
For example, a financial dataset might include technical structure details, but business annotations explain how metrics are calculated, why they matter to specific business processes, and any cautions about interpreting them.
Advanced search and discovery capabilities
Advanced search in modern data catalogs goes beyond keywords to include semantic search, faceted navigation, and relevance ranking. These features help users quickly find relevant data in complex environments where browsing would be impractical.
Key search and discovery features include:
- Natural language queries
- Semantic search understanding context and intent
- Faceted navigation for filtering results
- Recommendation engines suggesting relevant datasets
- Personalized results based on user roles and preferences
These capabilities dramatically reduce the time spent finding appropriate data. Industry estimates show data professionals typically spend 50% or more of their time just locating and accessing the right data. Effective search in data catalogs can significantly improve this efficiency.
A marketing analyst looking for customer segmentation data could simply type "Find recent customer purchase data for the West region" and quickly get relevant datasets, along with related analyses and reports.
Integration with data transformation tools
Leading data catalogs integrate with data transformation and analytics tools to create seamless workflows from discovery to insight. This integration lets users move directly from finding data to analyzing it without switching systems or manually transferring information.
Key integration patterns include:
- Embedded catalog views within analytics tools
- One-click data access from catalog to transformation tool
- Automatic lineage capture across the toolchain
- Metadata synchronization between catalog and data pipelines
This integration bridges the gap between discovery and action, which is essential for delivering value from data assets. By connecting the data catalog with tools used for data preparation, analysis, and visualization, organizations speed up insight generation and ensure consistency throughout the data lifecycle.
For instance, imagine a data scientist discovering a relevant dataset in the data catalog, then with a single click, opening that dataset in a Jupyter notebook for analysis, with all the necessary context and lineage information readily available.
By implementing these key components, organizations transform their data catalogs from passive repositories into active enablers of data-driven decision-making. This evolution supports business growth by making data more accessible, understandable, and actionable across the entire organization.
Types of tools for effective data cataloging
The data catalog landscape offers diverse tools designed for different organizational needs and maturity levels. Let's explore the main categories of data catalog tools and what makes each unique.
Open-source data catalog solutions
Open source data catalogs offer flexibility, community support, and cost-effectiveness. They're ideal for organizations with strong technical teams or specific customization needs, providing a foundation for tailored catalog environments.
Key advantages include:
- Flexibility to modify and extend functionality
- Active community support and contributions
- Lower upfront costs compared to commercial solutions
The catch? Open source options often require more in-house expertise for implementation and maintenance. While the software itself costs nothing, consider the total ownership cost, including resources needed for customization, integration, and ongoing support.
Popular open-source data catalog examples include Apache Atlas, built for Hadoop environments with strong lineage capabilities, Amundsen, created by Lyft, focusing on search and discovery with a modern interface, DataHub, LinkedIn's metadata platform emphasizing scalability and extensibility, and CKAN, a mature solution widely used in open data initiatives and government sectors
These solutions offer robust functionality but typically require significant technical resources to implement and maintain compared to commercial alternatives.
Cloud-native data catalogs
Cloud-native data catalogs work seamlessly with modern cloud data platforms, offering deep integration and scalability. They're perfect for organizations using cloud data warehouses and lakes.
For Databricks users, cloud-native data catalogs offer distinct advantages:
- Direct integration with Databricks' Unity Catalog
- Automatic capture of table metadata, notebook lineage, and usage patterns
- Ability to surface catalog information within the Databricks interface
These data catalogs sync automatically with changes in your cloud environment, keeping metadata current without manual work. This tight integration reduces administration and provides consistent governance across cloud and on-premises data.
The business benefits include faster time-to-value, less maintenance, and better data visibility across hybrid and multicloud environments.
AI and machine learning-enhanced data catalogs
AI and machine learning are transforming data catalogs from passive repositories into intelligent assistants, much like they are enhancing ETL processes for data leaders. According to our recent survey of data leaders, data transformation and preparation (34%) will see the most significant impact from applying GenAI, followed closely by data quality (30%).
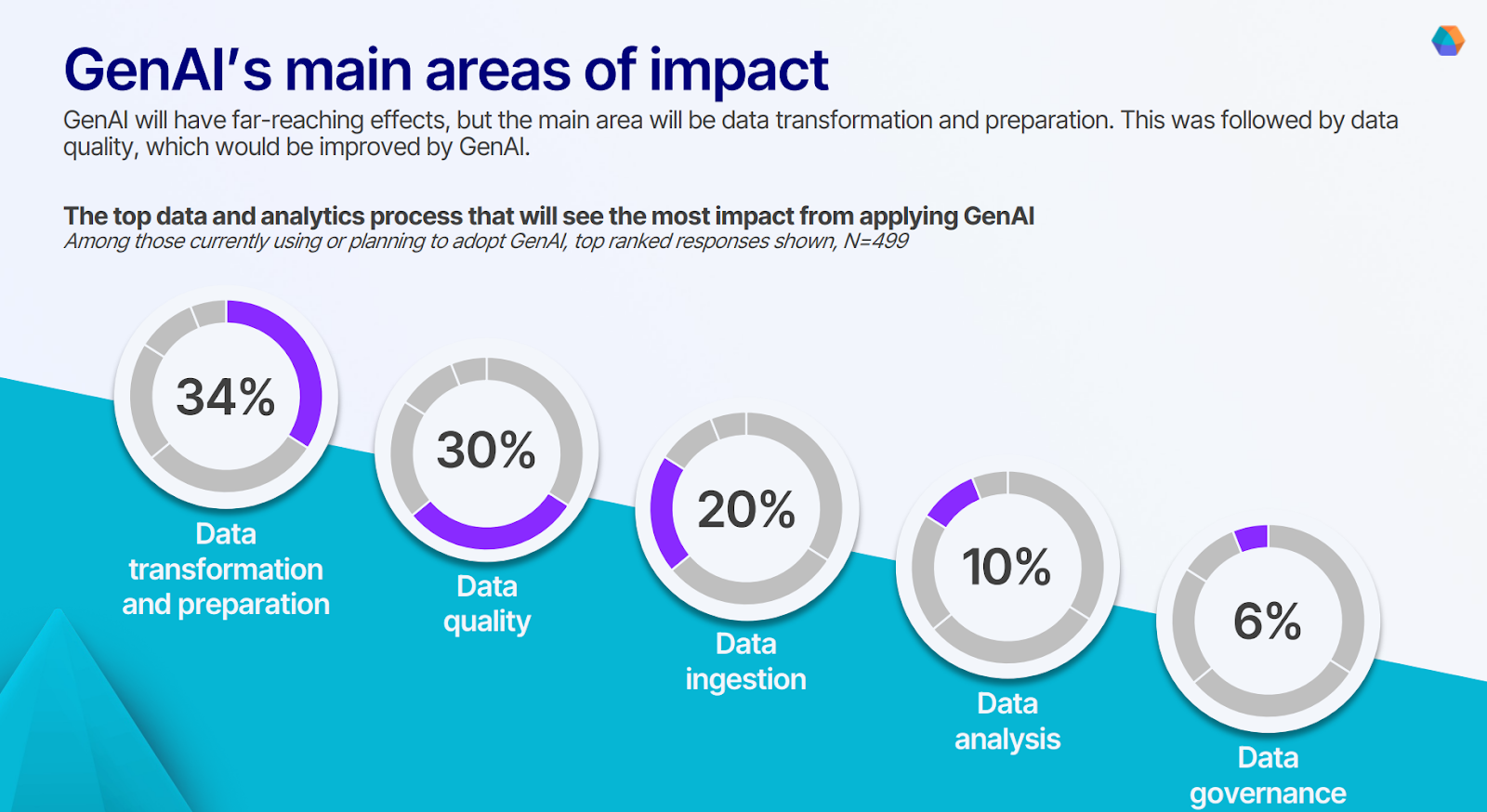
This aligns perfectly with how AI is revolutionizing data catalogs—automating complex transformation processes and improving quality assessments that previously required extensive manual effort.
Key AI-driven features include:
- Automated tagging and classification of data assets
- Intelligent data quality assessments
- Recommendation engines for relevant datasets
- Natural language processing for improved search
AI-enhanced data catalogs solve critical challenges like maintaining accuracy at scale and personalizing the discovery experience for different users. By automating tedious tasks like tagging and classification, they reduce administrative work while improving the user experience.
An AI-powered data catalog might automatically identify sensitive data fields, suggest relevant datasets based on your role and past behavior, or let you search for data using conversational language.
How to choose the right data cataloging tool
Selecting the right data catalog solution requires careful consideration of several factors. Start with clear requirements gathering, involving stakeholders from both IT and business units. This helps ensure the chosen data catalog aligns with organizational goals and user needs.
When selecting a data catalog solution, be careful to avoid common pitfalls in the evaluation process. Focusing exclusively on technical features without considering adoption factors can lead to sophisticated tools that go unused.
Similarly, prioritizing immediate needs without accounting for future growth may result in solutions that quickly become inadequate as your data ecosystem expands. Many organizations also underestimate the resources required for proper implementation and ongoing maintenance, which can significantly impact the total cost of ownership and the overall success of your data catalog initiative.
Remember: the most effective data catalog is one that people actually use and that delivers measurable business outcomes. A tool with advanced features may fail if it's too complex, while a simpler solution that everyone adopts can drive significant value.
Power data integration after data cataloging
Despite advancements in data cataloging, organizations still struggle with the "last mile" problem—turning discovered data into actionable business outcomes.
Roberto Salcido, System Architect at Databricks, emphasizes that "When business users can access and transform data through low-code interfaces, they're able to act on the insights discovered much more quickly." This critical transformation stage is where many data initiatives stall, leaving valuable cataloged assets underutilized.
Prophecy’s self-service with governance approach applies equally to both cataloging and integration, enabling organizations to fully leverage their data assets through low-code approaches.
Here's how Prophecy enables governed self-service data integration that complements data cataloging:
- Prophecy provides an intuitive visual interface for creating data pipelines. This allows business users to design and implement complex transformations without writing code, accelerating the journey from data discovery to insight.
- As users build pipelines visually, Prophecy automatically generates optimized code in the background. This ensures that transformations are efficient and scalable, while still maintaining the ease of use for non-technical users.
- Prophecy integrates natively with Databricks, allowing users to leverage the power of the Databricks platform for data processing. This tight integration ensures that data catalog information flows seamlessly into the transformation process.
- The platform supports team-based development with features like version control, code reviews, and shared workspaces. This fosters collaboration between data engineers, analysts, and business users, much like modern data catalogs do.
- Prophecy maintains detailed lineage information for all data transformations, complementing the lineage data in data catalogs. This end-to-end visibility is crucial for governance, auditing, and troubleshooting.
To bridge the critical gap between finding cataloged data and extracting actual business value, explore How to Assess and Improve Your Data Integration Maturity to transform how your organization leverages its data assets.
Ready to give Prophecy a try?
You can create a free account and get full access to all features for 21 days. No credit card needed. Want more of a guided experience? Request a demo and we’ll walk you through how Prophecy can empower your entire data team with low-code ETL today.
Ready to see Prophecy in action?
Request a demo and we’ll walk you through how Prophecy’s AI-powered visual data pipelines and high-quality open source code empowers everyone to speed data transformation
Get started with the Low-code Data Transformation Platform
Meet with us at Gartner Data & Analytics Summit in Orlando March 11-13th. Schedule a live 1:1 demo at booth #600 with our team of low-code experts. Request a demo here.